Elucidate Learning Principles With Mathematics
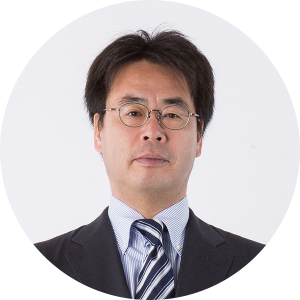
- NOBORU MURATA
- Professor, Department of Electrical Engineering and Bioscience /
Department of Advanced Science and Engineering,
Graduate School of Advanced Science and Engineering, Waseda University
The brain has rich ability and flexibility to perform various types of information processing. Information distributed in the brain is processed in parallel over a large number of mutually connected neurons. There must be mathmatical principles of parallel and distributed information processing, and the brain can be regarded as a biological realization of these principles as a result of evolution. Recently, many researchers have been interested in modeling information processing of learning systems. Sometimes models are simplified reproductions of the biological neural systems, but sometimes they are far from the real brain.
Even if models are different from the actual brain, they could be an engineering realization of the above mentioned information principles that the brain also uses in different styles. From this point of view, it is important to elucidate mathematical principles of learning systems and will be useful in various fields of engineering sciences, such as control theory, pattern recognition, energy management optimization and material design.
A necessary prerequisite for constructing machines that can solve application tasks is a solid theory of learning. We have used so far two very powerful and successful techniques for the analysis of learning machines to solve a number of problems: stochastic modeling and geometry of probability distributions.
When the data include noise, the input-output relation is described stochastically in terms of the conditional probability. Some learning machines are stochastic in their own nature, and hence their behaviors are also described by probability distributions and stochastic dynamics. Even when a machine is deterministic, it is effective to train it as if it were a stochastic machine, although it behaves deterministically in the execution mode. Adopting this point of view, we can treat learning machines within the framework of statistics and probability theory, and it gives great deal of advantages for analyzing their properties.
Another important theory originates from geometry. Analysis of the behavior of single learning machines have been carried out by many researchers using different mathematical methods and computer simulations. However, it has turned out to be a very successful technique to study not a specific machine but a whole family of machines to clarify their capabilities and limitations of a fixed architecture. Considering a learning machines including n-dimensional modifiable parameter, the set of all the possible machines realized by changing their parameters forms an n-dimensional manifold, where the set of parameters plays the role of a coordinate system. The geometry of this manifold is useful for understanding the total capability of a class of learning machines. This trial that connects the stochastic and geometrical ideas starts in 1980’s and it is called information geometry. It originates from the information structure of a manifold of probability distributions and has been developed to be a new mathematical subject with new differential geometrical notions, and it has been successfully applied to analyzing properties of learning machines.
NOBORU MURATA
Professor, Department of Electrical Engineering and Bioscience /
Department of Advanced Science and Engineering,
Graduate School of Advanced Science and Engineering, Waseda University
Program member
Mathematical engineering
- KEYWORD
- Mathematical engineering
Machine learning
Statistical learning theory
- Biography
- [EDUCATIONAL HISTORY]
1987 : Bachelor in Engineering, University of Tokyo, Tokyo, Japan
1989 Master in Engineering, University of Tokyo, Tokyo, Japan
1992 Doctor in Engineering, University of Tokyo,Tokyo, Japan
[EMPLOYMENT HISTORY]
1996 Research Associate Department of Mathematical Engineering and Information Physics,University of Tokyo
1995 - 1996 Visiting Researcher
1996 GMD FIRST, Berlin (the Research Institute for Computer Architecture and Software Technology of German National Research Center for Information Technology)supported by Alexander von Humboldt Foundation
1997 Frontier Researcher Lab for Information Representation, Brain Information Processing Group, the Institute of Physical and Chemical Research (RIKEN) Researcher
1997 -2000 Lab for Information Synthesis, Brain-Style Information System Group,RIKEN Brain Science Institute.
2000 - 2005 Associate Professor Department of Electrical, Electronics, and Computer Engineering,Waseda University
2005 - present ProfessorDepartment of Electrical Engineering and Bioscience,
Waseda University
- Related links
- OFFICIAL PAGE
- Contact
-
*e-mail will be delivered to teaching staff
*Please fill out all required items before sending